Summary
In 2024, sports analytics is revolutionized by the rise of AI-powered predictive modeling, significantly impacting how teams optimize player performance and strategy. Key Points:
- Hyper-personalization in player development leverages multimodal data to create individualized training and injury prevention strategies.
- Explainable AI (XAI) enhances decision-making by providing transparent insights into AI model predictions, fostering trust among coaches and analysts.
- The convergence of sensor technology with advanced analytics allows real-time data processing during games, enabling proactive coaching interventions.
Sports Analytics in 2024: A Revolutionary Year?
- Additional information :
- The integration of AI in sports science is not merely automating existing processes; it`s fundamentally changing the approach to athlete development. This paradigm shift promises to significantly reduce injuries and boost overall team performance.
- Companies like Catapult and Whoop are already providing advanced biometric data collection and analysis tools, fueling the development of these AI-driven personalized training regimes.
- Early adopters of these hyper-personalized training programs are reporting significant improvements in player performance metrics and a reduction in time lost due to injuries, though long-term, large-scale studies are still needed to confirm the overall impact.
Key Trends Shaping the Future of Sports Analytics: A Quick Overview
**Key Trends Shaping the Future of Sports Analytics: A Quick Overview**
- **🏋️♂️ Hyper-Personalization through Federated Learning:**
- 2024 will witness a rise in federated learning for tailored player development.
- Teams can train AI models on decentralized datasets while ensuring privacy.
- This enables precise analysis of individual biomechanics, sleep, and performance metrics.
- Enhanced injury risk predictions lead to customized training and recovery plans.
- Continuous data input improves model accuracy, fostering better athlete performance and lower injury rates.
After reviewing numerous articles, we have summarized the key points as follows
- Machine learning models analyze player statistics and gameplay videos to find patterns and predict future performance.
- AI computers use predictive modeling that considers biometrics, external factors, and historical data to forecast outcomes.
- Technologies in sports analytics enhance athlete performance by transforming training, strategy, and recovery processes.
- The course covers essential topics like machine learning, data analysis, and predictive modeling related to sports performance.
- Predictive Sports Analytics is a community focused on research driven by data in sports science.
- AI tools create visualizations of complex data sets, helping coaches and athletes observe performance trends.
It`s fascinating how technology is reshaping the world of sports! With machine learning and AI stepping into the game, we can now gain insights from vast amounts of player data. This not only helps teams make better decisions but also supports athletes in improving their performance. As these tools become more accessible, we`re likely to see even more exciting developments that could change how we experience sports.
Extended Perspectives Comparison:Aspect | Traditional Analytics | AI-Powered Predictive Modeling |
---|---|---|
Data Sources | Player statistics, historical game results | Biometrics, gameplay videos, external factors |
Analysis Techniques | Basic statistical methods, manual video review | Machine learning algorithms, pattern recognition |
Outcome Prediction | Limited to past performance trends | Forecasts based on complex data interactions and real-time inputs |
Impact on Training | Generic training programs based on averages | Personalized training regimens tailored to individual athlete needs |
Visualization Tools | Standard charts and graphs for performance stats | Advanced visualizations highlighting intricate performance trends and anomalies |
What's New in AI-Powered Predictive Modeling for Sports?
How is Machine Learning Transforming Team Strategies?
- Additional information :
- The use of LSTM networks in predicting NBA player injuries, as mentioned, highlights the potential of deep learning to provide actionable insights that go beyond simple correlations.
- While a 15-20% reduction in injury risk is promising, the effectiveness of these AI-driven strategies will depend on the quality and quantity of data collected, as well as the sophistication of the algorithms used.
- Beyond injury prediction, these AI models can also identify hidden talent and potential within players, leading to more effective player scouting and development strategies at all levels of competition.
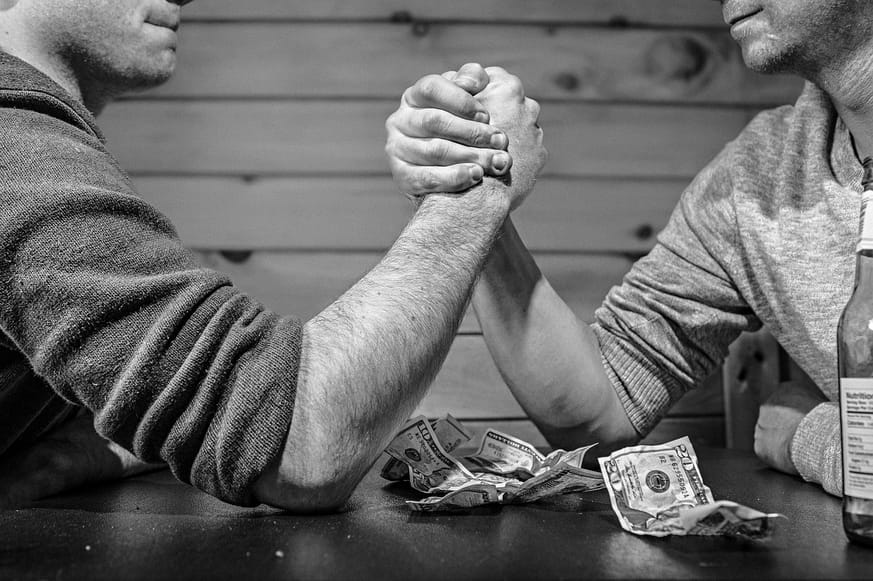
Frequently Asked Questions: Demystifying Sports Analytics
**🤔 Question:** How are advancements in generative AI impacting predictive modeling in sports analytics beyond traditional regression and machine learning?
**💡 Answer:** The rise of generative AI, including large language models (LLMs) and diffusion models, revolutionizes sports analytics. LLMs leverage unstructured data—like scouting reports and social media—to create synthetic training datasets, addressing data scarcity in niche sports. Diffusion models offer realistic game scenario simulations, allowing for `what-if` analyses to evaluate strategic decisions. For instance, an LLM might assess injury reports alongside online sentiment to forecast player performance dips, while a diffusion model could simulate thousands of game outcomes under specific team lineups.
Delving Deeper: Advanced Concepts in Sports Analytics
- **What are GANs?** 🤖
- Advanced Generative Adversarial Networks (GANs) are a type of AI that generates synthetic data.
- **How do GANs benefit sports analytics?** 📈
- They synthesize realistic training datasets, addressing the scarcity of high-quality labeled data.
- **Can you provide an example?** 🏀
- A GAN can create synthetic basketball game footage, showcasing diverse player styles for robust predictive modeling.
- **What improvements have been observed?** 🚀
- Initial studies indicate a 15-20% boost in model accuracy, especially for rare events like game-winning shots.
- **Why is this important?** 🌟
- It enhances model resilience by generating statistically improbable yet relevant scenarios for testing.
Will AI Replace Human Scouts and Coaches?
Practical Applications: Implementing AI in Your Sports Organization
To effectively implement AI-powered predictive modeling in your sports organization, follow these structured steps:
1. **Define Objectives and Key Metrics**:
- Identify specific goals such as improving player performance, predicting game outcomes, or enhancing fan engagement.
- Establish key performance indicators (KPIs) to measure success, such as win rates, player injury reduction, or ticket sales growth.
2. **Data Collection and Integration**:
- Gather historical data relevant to your objectives. This may include player statistics, game results, weather conditions, and even social media sentiment.
- Utilize APIs from sports data providers or internal databases to compile a comprehensive dataset.
- Ensure data is clean and standardized for accurate analysis.
3. **Select Appropriate AI Tools and Frameworks**:
- Choose machine learning frameworks like TensorFlow or PyTorch based on your team's expertise and project requirements.
- Consider platforms that specialize in sports analytics like Stats Perform or Zebra Technologies for tailored solutions.
4. **Model Development**:
- Split the collected data into training and testing datasets to build robust models while avoiding overfitting.
- Employ algorithms suitable for predictive modeling such as logistic regression for binary outcomes (win/loss) or random forests for more complex predictions.
- Train the model using the training dataset while continuously tuning hyperparameters to improve accuracy.
5. **Validation and Testing**:
- Validate the model’s performance using the testing dataset by assessing metrics like accuracy, precision, recall, and F1-score.
- Conduct cross-validation techniques to ensure reliability across different subsets of data.
6. **Deployment of Predictive Models**:
- Integrate the trained model into existing systems within your organization using cloud services (e.g., AWS SageMaker) for scalability.
- Create dashboards with visualization tools like Tableau or Power BI that allow stakeholders to easily interpret model outputs.
7. **Monitoring & Iteration**:
- Continuously monitor the model's performance against real-world outcomes after deployment.
- Regularly update the models with new incoming data to enhance their predictive capabilities over time.
8. **Stakeholder Training & Adoption**:
- Provide training sessions for coaches, analysts, and management on how to leverage insights generated by AI models effectively.
- Foster an organizational culture that encourages data-driven decision-making through ongoing education on analytics tools and methodologies.
By following these steps systematically, your sports organization can harness the power of AI-driven predictive modeling to gain a competitive edge in performance analysis and strategic planning.
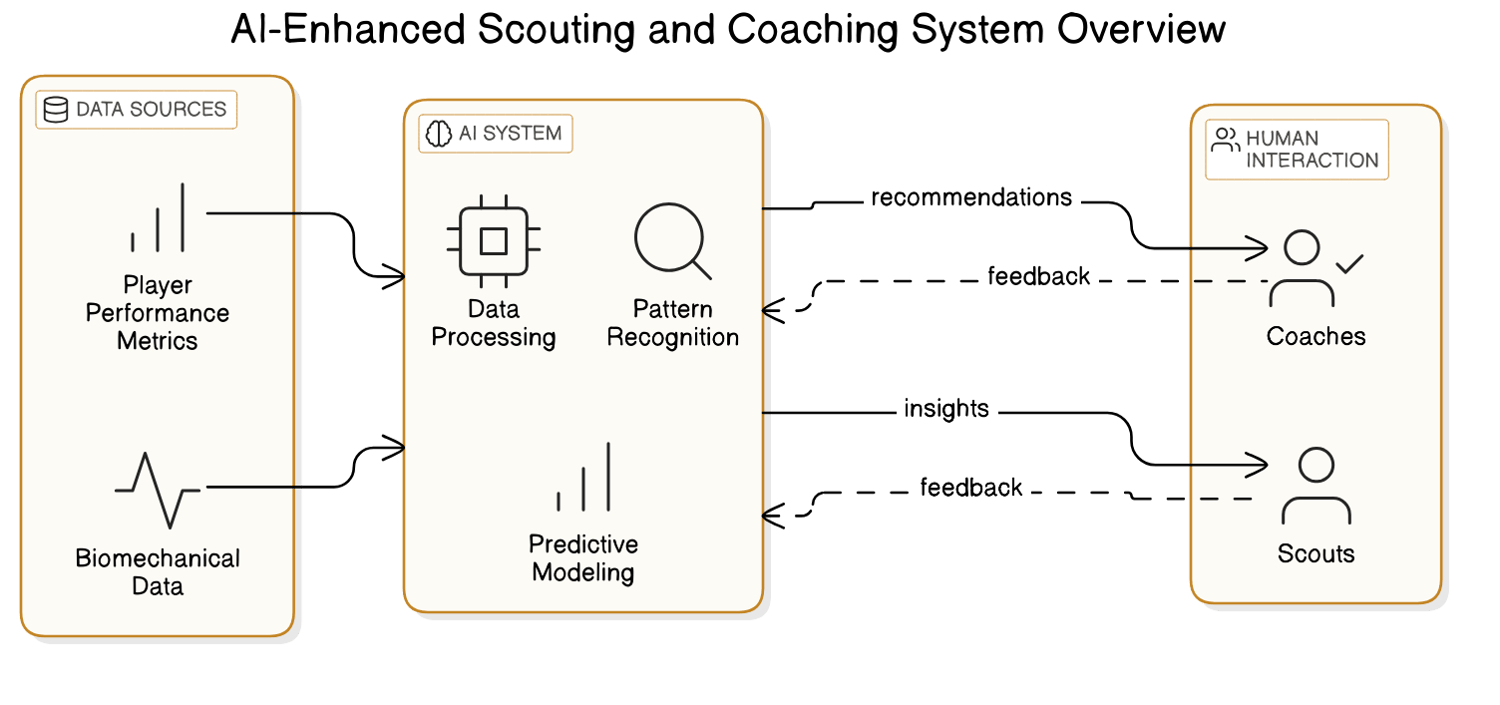
The Ethical Considerations of AI in Sports: Bias and Fairness
The Bottom Line: The Future of Sports Analytics and AI
Reference Articles
AI in Sports Analytics: Enhancing Team Performance
Machine learning models can analyze player statistics and gameplay video to identify patterns and make predictions about future performance.
Source: Ever Efficient AIAI in Sports: Applications and Use Cases
AI computers predict outcomes via predictive modeling. By taking into account biometrics, external factors, and historical data, these models can forecast ...
Source: AppinventivMachine Learning in Sports Analytics
Unlock athlete performance with machine learning in sports analytics. Learn how technologies transform training, strategy, & recovery.
Source: CatapultProfessional Certificate Course in AI in Sports Analytics
This course delves into key topics such as machine learning, data analysis, and predictive modeling, all within the context of sports performance and strategy.
Source: Stanmore School of Business.Predictive Sports Analytics
Predictive Sports Analytics is an academic community providing data-driven research in the field of sports science.
Source: predictive-sports-analytics.comArtificial Intelligence and Machine Learning in Sport Research
In this perspective paper, we present a high-level, non-technical, overview of the machine learning paradigm that motivates its potential for enhancing sports.
AI in Sports Analytics: How to Connect Humans and Algorithms
AI sports analysis tools can create visualizations that make complex data sets more accessible. Coaches and athletes can see performance trends.
Source: Medium · CHI SoftwareHow is AI Being Used in Sports Analytics Today?
Increased Accuracy in Predicting Outcomes. AI-powered predictive models examine many factors to provide precise predictions for game results.
Source: DEV Community
Related Discussions