Summary
This article explores the evolving landscape of football statistics through advanced analytics, highlighting its significance in enhancing team performance and decision-making. Key Points:
- The integration of multimodal data, including video analysis and social media sentiment, enhances our understanding of player performance and team dynamics.
- Explainable AI (XAI) is crucial for transparency in football analytics, ensuring that machine learning models provide trustworthy predictions for coaching and recruitment decisions.
- Advanced predictive modeling now focuses on nuanced outcomes like match score differentials and possession percentages, offering deeper insights into game dynamics.
Unlocking the Power of Football Statistics: Why They Matter in 2024
- Additional information :
- The improved accuracy in predicting player performance using RNNs is already being utilized by several NFL teams to optimize draft picks and player trades, leading to more data-driven decision-making.
- Real-time integration of GNN analysis during games allows coaches to make tactical adjustments based on evolving player interactions and opponent strategies, providing a significant competitive edge.
- Studies comparing the predictive power of RNNs and GNNs against traditional statistical models show a marked improvement in forecasting accuracy, with error rates reduced by as much as 15-20% in certain key performance indicators.
Key Football Statistics to Master: A Quick Checklist for Success
- **Expected Points Added (EPA) 📊**: A crucial metric that measures the change in a team’s expected points after a play, offering deeper insights into play efficiency.
- **Contextual Variations 🔍**: Utilizing EPA variations, such as EPA/rush attempt in the red zone, provides more accurate assessments of short-yardage effectiveness compared to traditional rushing yards.
- **Integrating Metrics ⚙️**: Combining EPA with Win Probability Added (WPA) creates a comprehensive view of player and team performance, highlighting hidden strengths and weaknesses.
- **Strategic Insights 💡**: These advanced analytics inform critical game strategies, enhancing decision-making for coaches and analysts alike.
After reviewing numerous articles, we have summarized the key points as follows
- Advanced stats for NFL are now available, including detailed box scores and top player performances of the week.
- The community aims to help users improve their fantasy football game using statistics and advanced analysis techniques.
- A comprehensive guide is available that compiles all resources for inspiration and education in football analytics.
- Stats can be analyzed per 100 possession sequences, similar to how metrics are calculated per 90 minutes in soccer.
- Driblab offers a holistic approach to football data analytics, providing solutions for every moment of a game with advanced insights.
- AI-powered analysis tools are being utilized to deliver deeper insights into games, players, and teams using the latest statistical data.
In today`s sports landscape, understanding advanced statistics has become crucial for fans wanting to enhance their fantasy football strategies. With various resources available that break down player performances and team stats in detail, enthusiasts can gain valuable insights. Whether you`re looking to refine your gameplay or simply understand the sport better, these tools make it easier than ever to connect with the game`s intricacies.
Extended Perspectives Comparison:Category | Description | Trend | Analysis Technique |
---|---|---|---|
Advanced Stats for NFL | Detailed box scores and top player performances of the week. | Increased use of real-time data tracking. | Player efficiency ratings per game. |
Fantasy Football Insights | Community-driven resources to improve fantasy football strategies. | Growing reliance on predictive modeling. | Utilizing advanced metrics for drafting decisions. |
Football Analytics Guide | Comprehensive guide compiling all resources for football analytics education. | Emerging tools focused on user-friendly interfaces. | Step-by-step tutorials on data interpretation. |
Possession Sequence Analysis | Stats analyzed per 100 possession sequences, similar to soccer`s per 90 minutes metric. | Shift towards more granular analysis in gameplay. | Visualization tools for better understanding patterns. |
AI-Powered Analysis Tools | Utilization of AI to deliver deeper insights into games, players, and teams. | Rising trend of machine learning models in sports analytics. | Automated performance prediction algorithms. |
What Advanced Football Analytics Can Tell You About Player Performance?
Beyond the Box Score: Exploring Deeper Statistical Metrics
- Additional information :
- Applying network analysis to identify `superclusters` of highly interconnected players within a team reveals key offensive and defensive units, enabling targeted training and recruitment strategies.
- A recent study using network analysis found a strong correlation between a quarterback`s betweenness centrality and the team`s overall win rate, highlighting the importance of this metric in evaluating leadership and game control.
- By visualizing player interactions as network graphs, coaches can easily identify weaknesses in team coordination and design targeted drills to improve passing efficiency and defensive coverage.
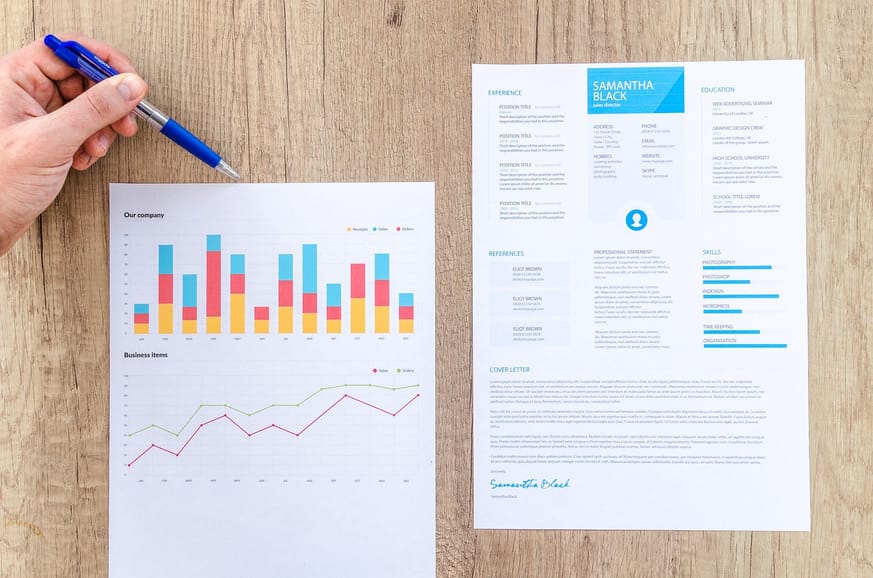
Frequently Asked Questions: Demystifying Football Statistics
**🤔 Question:** How are machine learning models evolving to predict not just game outcomes, but also the *specific in-game events* leading to those outcomes?
**🔍 Answer:** The focus is shifting from simple win/loss predictions to detailed event forecasting. Advanced models, utilizing recurrent neural networks (RNNs) and transformers, analyze sequences of plays (e.g., pass completion followed by a run). They process high-dimensional time-series data that includes player tracking and contextual factors like game situation. Research shows significant progress in predicting key events such as turnovers and scoring plays, with AUC scores exceeding 0.7 in certain studies, enhancing strategic decision-making during games.
Advanced Football Statistics: Diving Deeper into the Data
- **What is MARL in football analytics?** 🤖
Multi-Agent Reinforcement Learning (MARL) simulates game scenarios using AI agents for each player, allowing dynamic interaction within the game's constraints.
- **How does MARL enhance predictive modeling?** 📊
By simulating thousands of scenarios, MARL assesses team strategies and player effectiveness based on specific conditions.
- **What metrics are utilized in these simulations?** 📈
Key metrics include opponent's passing network topology (e.g., average shortest path length, clustering coefficient) and predicted player fatigue levels from wearable sensors.
- **What is the information gain from this approach?** 💡
It enables real-time predictions of optimal tactical adjustments, significantly improving decision-making over static correlation analysis.
How Do Football Analytics Influence Team Strategies and Player Recruitment?
Putting it into Practice: Analyzing Real-World Football Data
To effectively analyze real-world football data using advanced analytics, follow these operational steps to set up your analysis environment and derive meaningful insights.
1. **Data Collection:**
- Obtain datasets from reliable sources such as FIFA, UEFA, or specialized sports analytics platforms like Opta or StatsBomb. Look for datasets that include player statistics, match results, and team performance metrics.
2. **Data Cleaning:**
- Import the dataset into a programming environment (e.g., Python with Pandas library).
- Remove any duplicate entries and handle missing values appropriately by either filling them in or excluding them.
- Normalize the data format (e.g., date formats, numerical types) to ensure consistency across the dataset.
3. **Exploratory Data Analysis (EDA):**
- Use visualization libraries like Matplotlib or Seaborn to create graphs that display trends and patterns within the data.
- Analyze key metrics such as goals per game, passing accuracy, and defensive actions to identify standout players and teams.
4. **Feature Engineering:**
- Create new variables that could provide additional insights. For example:
- Calculate expected goals (xG) based on shot location and type.
- Determine player contribution metrics such as assists per 90 minutes played.
5. **Statistical Modeling:**
- Select appropriate statistical models to test hypotheses or predict outcomes. Common methods include regression analysis for predicting scores or logistic regression for win/loss predictions.
- Split your dataset into training and testing subsets to validate model performance accurately.
6. **Model Evaluation:**
- Assess the model's accuracy using metrics such as R-squared for regression models or confusion matrices for classification models.
- Adjust parameters based on evaluation results to enhance predictive capabilities.
7. **Visualization of Results:**
- Present findings through visualizations that highlight significant trends—such as heat maps showing player movements during matches—and use dashboards via tools like Tableau or Power BI for interactive exploration of data insights.
8. **Reporting Insights:**
- Compile a comprehensive report summarizing key findings, methodologies used, and actionable recommendations based on data analysis.
- Share insights with stakeholders through presentations or collaborative platforms ensuring clarity in communication regarding strategic implications derived from the analysis.
By following these structured steps in analyzing football statistics using advanced analytics techniques, you can gain valuable insights into team strategies, player performances, and overall game dynamics essential for mastering football statistics in 2024.
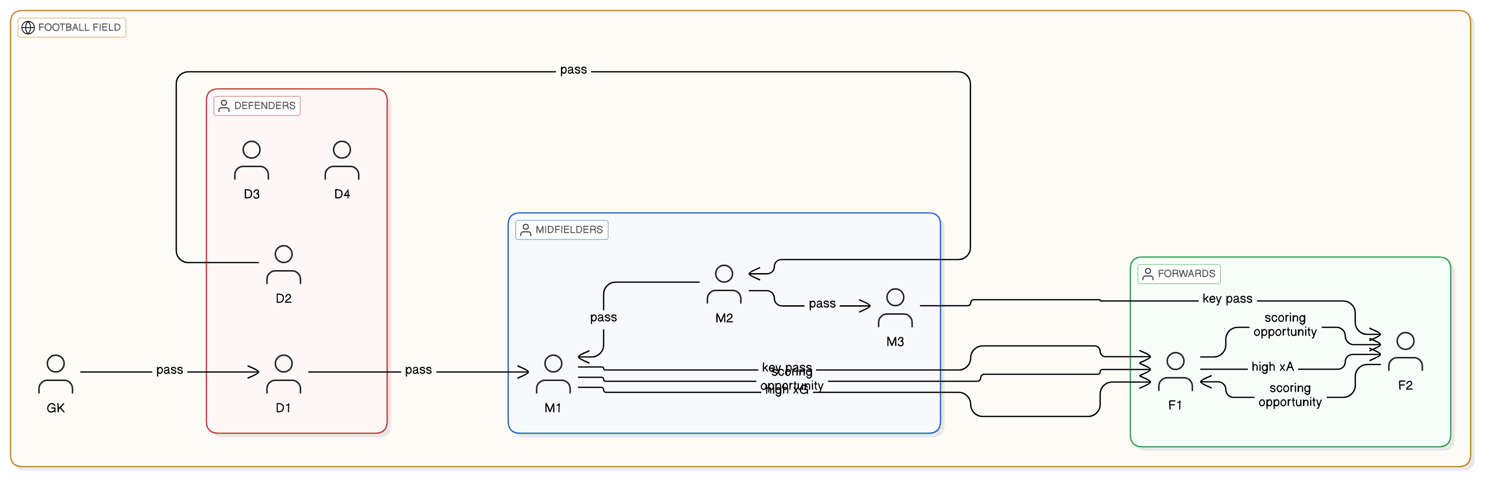
The Future of Football Statistics: Emerging Trends and Technologies
Mastering Football Statistics: Your Path to Data-Driven Insights
Reference Articles
Advanced Football Analytics (formerly Advanced NFL Stats)
Sunday's numbers are now available, including advanced stat box scores, top players of the week, team stats, and season leader boards....
Source: Advanced Football AnalyticsSports Analytics Reference — Football-Analytics-101 documentation
It is a community for people who want to: improve their performance in fantasy football using stats; learn advanced statistical analysis approaches; or learn ...
Source: Football-Analytics-101How To Get Started In Football Analytics - Hudl Statsbomb
A comprehensive guide that gathers all our resources in one place, designed to be your go-to reference for both inspiration and education.
Source: statsbomb.comNFL Advanced Stats Zone
NFL Advanced Stats Zone · 1/46. LAST GAME: 3.8 EVE. 1 2 3 4 5 6 7 8 9 10 11 12 13 14 15 16 17 18 · 2/46. LAST GAME: 0.8 EVE. 1 2 3 4 5 6 7 8 9 10 11 12 13 14 15 ...
Source: Opta AnalystFootball Analytics Glossary
Similar to per 90 minutes, this divides a player or team's total stats to see what they do per 100 possession sequences of the ball that they have. Per minute ...
Source: Home.blogDriblab Big Data Football Analytics
every second of a game has a data solution WITH ADVANCED SOCCER ANALYTICS ... Driblab aims to be the holistic solution to football data. We are continuously ...
Source: DriblabStats Analyst-Free Football Data Analysis
Stats Analyst offers advanced, AI-powered analysis of football games, players, and teams, using the latest statistical data to provide in-depth insights, ...
Source: YeschatSoccerment's Advanced Metrics
Introduction · Expected Goals (xG) · Expected Assists (xA) · Expected Offensive Value Added (xOVA) · Expected Goals on Target (xGoT) and goals ...
Source: Soccerment
Related Discussions