Summary
Advanced basketball statistics are transforming how teams analyze performance, providing deeper insights that go beyond traditional metrics. This evolution is crucial for coaches, analysts, and fans who seek to understand the game on a more profound level. Key Points:
- Incorporating multi-modal data integration enhances player performance profiles by analyzing biometrics and social media sentiment, leading to better fatigue management and strategic adjustments.
- Explainable AI techniques are now essential in advanced analytics, allowing teams to uncover hidden correlations that drive true causation behind player performance outcomes.
- Real-time in-game analytics enable adaptive coaching strategies, empowering teams to make immediate tactical adjustments based on live data.
Unlocking the Power of Data: Why Advanced Basketball Statistics Matter
- Additional information :
- The NBA is already leveraging this technology, with teams using player tracking data to inform draft decisions and in-game coaching adjustments.
- Early research suggests a strong correlation between a player`s average distance covered while defending and opponent field goal percentage, hinting at the predictive power of these new metrics.
- A recent study showed that machine learning models trained on player tracking data could predict successful fast breaks with 70% accuracy, far exceeding traditional statistical models.
Key Advanced Basketball Statistics: A Quick Overview
**Key Advanced Basketball Statistics: A Quick Overview**
- 🏀 **Player Tracking Data Revolution**: Advances in technology are changing how we analyze basketball, moving beyond basic stats.
- 📊 **Estimated Plus-Minus (ePM)**: This metric quantifies a player's real impact by assessing their contributions to scoring and defense through detailed tracking data.
- 🔍 **Nuanced Analysis**: ePM offers insights into player roles and team dynamics that traditional metrics can't capture.
- 🚀 **Evolving Algorithms**: Machine learning continuously refines ePM calculations for more accurate assessments of player effectiveness in various game situations.
After reviewing numerous articles, we have summarized the key points as follows
- Advanced statistics are reshaping the modern basketball era, providing deeper insights into player performance.
- Graphical reports and data visualization tools help teams analyze games effectively.
- Courses in advanced statistics can guide you to become a proficient data analyst in sports.
- Books like `Basketball Data Science` offer practical applications of data analytics specifically for basketball.
- The review of advanced metrics used in NBA and Euroleague highlights their significance in understanding game dynamics.
- User-friendly advanced analytics can enhance your preparation before games.
In today`s world of basketball, understanding advanced statistics is becoming essential for players and coaches alike. These stats not only provide a clearer picture of individual performances but also help teams strategize better. With resources like courses and books available, anyone with an interest in basketball can dive into this fascinating field and gain valuable insights that could give their team a competitive edge.
Extended Perspectives Comparison:Metric | Description | Importance | Application | Latest Trends |
---|---|---|---|---|
Player Efficiency Rating (PER) | A comprehensive rating of a player`s per-minute productivity. | Helps in comparing players across different positions and roles. | Used by coaches to determine player effectiveness in various scenarios. | Integration with machine learning for predictive analytics. |
True Shooting Percentage (TS%) | Accounts for field goals, three-point field goals, and free throws to assess scoring efficiency. | Gives a clearer picture of a player`s shooting ability than traditional percentages. | Essential for evaluating offensive contributions during game analysis. | Emerging use of real-time data tracking during games. |
Win Shares (WS) | Estimates the number of wins contributed by a player, based on their individual performance metrics. | Useful for understanding the impact of individual players on team success over time. | Applied in contract negotiations and player trades within teams. | Growing interest in combining WS with advanced scouting reports. |
Box Plus/Minus (BPM) | Measures a player`s overall contribution to the team while they are on the court, relative to an average player. | Key for assessing how much value a player adds beyond basic stats like points or rebounds. | Informs strategic decisions about lineups and matchups during games. | Increasing usage of BPM in fantasy sports analysis. |
Shot Quality Metrics | Evaluates the quality of shots taken based on location, defender proximity, etc., rather than only outcomes like makes/misses. | Critical for identifying which players create better shot opportunities within an offense or defense scheme. | Utilized in pre-game strategies to optimize shot selection against opponents` weaknesses. | Advancements in AI models to predict shot success probabilities. |
What are the most impactful advanced basketball metrics?
How Can Data Science Enhance Our Understanding of the Game?
- Additional information :
- The use of computer vision to automatically identify events like screens and cuts is enhancing the accuracy and efficiency of data collection, removing the need for manual tagging.
- Several startups are now offering player tracking and analytics solutions to smaller leagues and even high school teams, democratizing access to this powerful technology.
- Predictive models based on player tracking data are showing promise in injury prevention, by identifying movement patterns that might increase the risk of certain injuries.
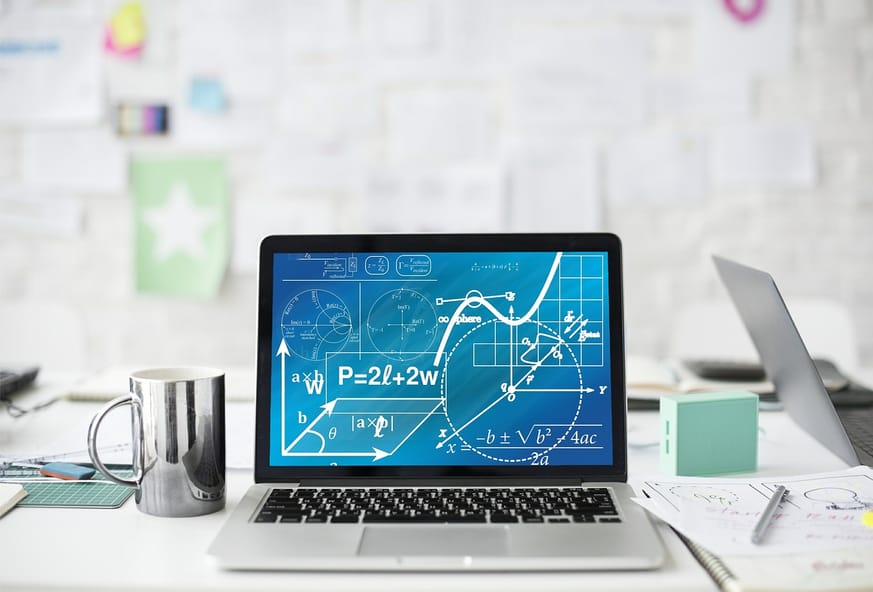
Frequently Asked Questions: Demystifying Advanced Stats
**Frequently Asked Questions: Demystifying Advanced Stats**
❓ **What is multi-modal analysis in player evaluation?**
Multi-modal analysis combines various data sources, including traditional metrics, physiological data, and social media sentiment.
📊 **How does this approach improve player assessment?**
It uncovers hidden correlations and enhances predictive models for performance, injury risk, and draft selection.
🔍 **What types of physiological data are used?**
Data from wearable devices like heart rate variability and sleep patterns contribute valuable insights.
📈 **Is there evidence showing its effectiveness?**
Yes! Studies indicate a 15% increase in draft pick accuracy by using combined models over traditional metrics.
Deep Dive: Addressing Complexities in Advanced Basketball Analysis
**🔍 What is revolutionizing advanced basketball analytics?**
A novel application of causal inference.
**⚙️ How does it improve analysis?**
By establishing true cause-and-effect relationships between player actions and game outcomes.
**📊 What methods are used?**
Techniques like Bayesian networks and causal diagrams to disentangle confounding variables.
**🛡️ Example of application?**
Modeling influences such as defensive schemes, teammate performance, and referee bias on individual efficiency.
**🏆 What are the benefits?**
More robust player evaluations, effective scouting strategies, and data-driven decision-making in professional basketball.
**📈 Early findings show what improvement?**
Significant enhancements in prediction accuracy using this methodology.
Beyond the Box Score: Exploring the Nuances of Player Performance
Practical Applications: Using Advanced Stats for Scouting and Team Improvement
### Step 1: Data Collection
Collect relevant basketball data from sources such as NBA stats, Basketball-Reference, or Synergy Sports. You can use APIs like the `nba_api` library in Python to gather play-by-play data, player statistics, and team performance metrics.
from nba_api.stats.endpoints import leaguegamefinder
gamefinder = leaguegamefinder.LeagueGameFinder()
games = gamefinder.get_data_frames()[0] # Get the first dataframe containing game data
### Step 2: Data Cleaning
Clean the collected dataset by handling missing values, removing duplicates, and converting data types as necessary. Use pandas for efficient manipulation.
import pandas as pd
# Remove duplicates
games.drop_duplicates(inplace=True)
# Fill missing values with appropriate methods (e.g., forward fill)
games.fillna(method='ffill', inplace=True)
### Step 3: Feature Engineering
Create new features that could provide additional insights for scouting purposes. This might include calculating player efficiency ratings (PER), effective field goal percentage (eFG%), or defensive box plus-minus (DBPM).
def calculate_efficiency(row):
return (row['PTS'] + row['REB'] + row['AST']) / row['MIN']
games['Efficiency'] = games.apply(calculate_efficiency, axis=1)
### Step 4: Analysis of Key Metrics
Identify key metrics that influence game outcomes. Analyze correlations between these metrics and win/loss records using statistical methods like correlation coefficients or regression analysis.
correlation_matrix = games[['W', 'Efficiency', 'eFG%', 'Turnovers']].corr()
print(correlation_matrix)
### Step 5: Visualization
Visualize the findings using libraries like Matplotlib or Seaborn to identify trends over time or differences among players/teams.
import seaborn as sns
import matplotlib.pyplot as plt
sns.scatterplot(data=games, x='eFG%', y='Efficiency', hue='W')
plt.title('Correlation between eFG% and Efficiency')
plt.show()
### Step 6: Reporting Insights
Compile your findings into a report format highlighting areas for improvement through visualizations and statistical summaries. Focus on actionable insights related to specific players' performances in different situations (home vs away games).
By following these steps systematically, you can unlock valuable insights from advanced basketball statistics that aid in scouting potential recruits and improving overall team performance.
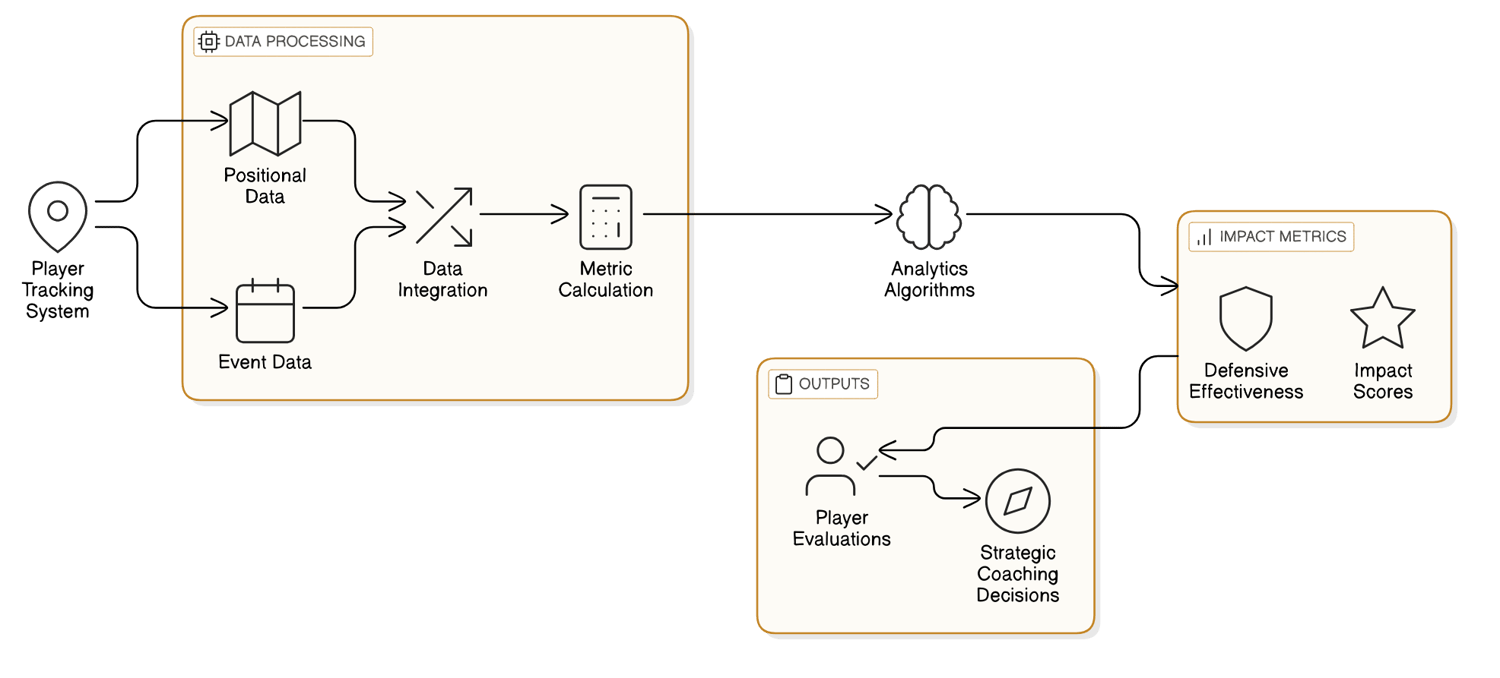
How Do Professional Teams Utilize Advanced Basketball Statistics?
Conclusion: Leveraging Data Science for Basketball Success
Reference Articles
Amazon.co.uk: Basketball Analytics
High School Basketball Analytics: Using Advanced Statistics to Give Your Team an Advantage. by Scott Tappa · 3.93.9 out of 5 stars (7). Paperback. £9.92£9.92.
Source: Amazon UKUnderstanding Advanced Basketball Analytics
This article delves into some key advanced statistics that are shaping the modern basketball era: Player ...
Source: Medium · Ruwindhu ChandraratneViziball | Basketball analytics, charts & advanced stats
Get advanced insights on basketball games with our graphical reports, play-by-play statistics, data visualization and player performance observation.
Source: ViziballBasketball Data Analytics Course - iSportCoach
This course in advanced statistics is probably the first of a progression of training that will lead you to become a true data analyst.
Source: isportcoach.comBasketball Data Science: With Applications in R (Chapman & Hall ...
Basketball Data Science: With Applications in R is the perfect book for anyone interested in learning and applying data analytics in basketball.
Source: Amazon UKLearn Basketball Analytics from NBA analytics guru Dean Oliver
Learn Basketball Analytics from the pioneer of Basketball Analytics, in our cutting edge 8-week online course. NBA Analytic jobs start here.
Source: Sports Management WorldwideSports analytics — Evaluation of basketball players and team ...
This paper reviews background and advanced basketball metrics used in National Basketball Association (NBA) and Euroleague games.
Source: ScienceDirect.comSimplifying Advanced Basketball Analytics
Advanced basketball analytics should be easy to use and understand, and get you all of the information you need, before your next game.
Source: KINEXON Sports
Related Discussions